Prompting is one of the most important techniques in getting the most out of a GPT (Generative Pre-trained Transformer) model as the quality and specificity of the prompt can significantly influence the output.
Effective prompting requires clear communication, context, and a good understanding of how to frame questions or tasks. A recent academic report called The Prompt Report: A Systematic Survey of Prompting Techniques examined how to do this best.
In this three-part series, we will look at the different types of prompting and provide examples of how you upgrade your prompting game to create content for your marketing needs.
Why is AI Prompting Important?
As AI technologies continue to mature, the sophistication of user interactions with these systems has significantly increased, particularly through optimized prompts. These prompts are not merely instructions but are carefully designed templates that guide AI to generate more accurate, relevant, and contextually appropriate responses.
This article delves into the nuances of creating effective prompts, focusing on multimodal prompts, which are prompts that integrate various data types, such as text, images, and audio, to enhance the AI’s understanding and output.
By exploring how to optimize these multimodal prompts, you get better outputs that can be directly applied to enhance customer engagement, improve content creation processes, and streamline operations within your brand.
Exploring the Different Types of Prompts
Here are eight different types of prompts. Each has its advantages and can be used based on the specific requirements of the task at hand.
1. Zero-Shot Prompts
Definition: Zero-shot prompting involves presenting a task to an AI model without any specific training or examples for that particular task.
Use Case: Useful when you need to assess the model’s baseline understanding or when you have a task where similar examples have yet to be shown to the model.
Advantages: Quick to deploy as it doesn’t require gathering examples; good for testing a model’s general capabilities.
Limitations: This may not yield the most accurate or optimal responses since the model relies solely on its pre-training.
Prompt Example: “Translate the following sentence into French: ‘Where is the nearest grocery store?'”
Context: This prompt tests the model’s ability to translate without prior examples of translation tasks given in the session.
2. Few-Shot Prompts
Definition: Few-shot prompts involve giving the AI model a few examples (or “shots”) to help it understand the context or type of output expected.
Use Case: Effective when the task is specific and the model needs a few examples to “learn” what is expected without full-scale training.
Advantages: Balances between zero-shot and fine-tuning by providing some guidance to improve accuracy without extensive training.
Limitations: The quality of the output can heavily depend on the quality and relevance of the examples provided.
Prompt Example: “Here are examples of emails categorized as ‘urgent’ or ‘non-urgent’. Classify the following email:
Example 1: Subject: Meeting Today – Urgent
Example 2: Subject: Weekly Newsletter – Non-Urgent
New Email: Subject: Immediate Response Required – [classify this]”
Context: This prompt helps the model understand what criteria might define ‘urgent’ vs. ‘non-urgent’ emails.
3. Chain-of-Thought (CoT) Prompts
Definition: Chain-of-thought prompting requires the model to articulate intermediate steps or reasoning paths leading to its conclusion.
Use Case: Valuable for tasks involving complex reasoning or when you need transparent, explainable AI outputs.
Advantages: Can significantly enhance model performance in logical reasoning and complex problem-solving tasks.
Limitations: More verbose outputs which may not always be necessary, and the process might be slower due to the detailed reasoning required.
Prompt Example: “A baker used 25% of a flour sack. If the sack originally contained 20 pounds of flour, calculate how much flour remains. Start by explaining how much flour was used.”
Context: This prompt guides the model through a step-by-step calculation process, enhancing its ability to solve the problem accurately.
4. In-Context Learning
Definition: In-context learning prompts involve positioning the question or task within a context that the model can use to infer the correct response.
Use Case: Useful for tasks where additional background information is essential for generating accurate and relevant responses.
Advantages: Helps the model make more informed decisions and improves the relevance of the responses.
Limitations: Requires carefully crafted prompts to ensure the context is relevant and not misleading.
Prompt Example: “Given the context of increasing urban air pollution, discuss the potential benefits of electric vehicles in reducing environmental impact.”
Context: This prompt sets a specific scenario that helps the model generate a relevant and informed response based on the given background.
5. Meta-Prompting
Definition: Meta-prompting involves using prompts to generate or refine other prompts, essentially using the model to improve its own instructions.
Use Case: Can be employed in automated systems where continuous learning and adaptation of prompts are needed without human intervention.
Advantages: Leads to dynamic improvement of prompting strategies, adapting to new data or tasks efficiently.
Limitations: Might lead to complex recursive loops or inefficient prompt generation without proper constraints.
Prompt Example: “Generate a prompt that would ask for a summary of a scientific paper’s methodology.”
Context: This prompt asks the AI to create another useful prompt, which is particularly effective in automated systems for generating user-specific queries.
6. Multimodal Prompts
Definition: These prompts combine different types of data (e.g., text, images, audio) to guide the AI’s response.
Use Case: Useful in scenarios where the integration of multiple data types can lead to a richer understanding of the task, such as in content creation for social media.
Advantages: Enhances the model’s ability to handle complex inputs and outputs that require understanding beyond plain text.
Limitations: Requires models specifically trained to handle and integrate multiple data types effectively.
Prompt Example: [Image of a crowded city street] + “Describe the traffic conditions shown in this image and suggest improvements for better flow.”
Context: This prompt combines visual data with a text question, challenging the model to analyze the image and apply textual reasoning to provide solutions.
Companies interested in learning more about how to adopt AI in their business can contact Zozimus. With our expertise in AI technologies and prompt optimization, we can help you harness the power of AI to transform your operations, improve customer engagement, and drive innovation. Reach out to us today to explore how AI can elevate your business to new heights.
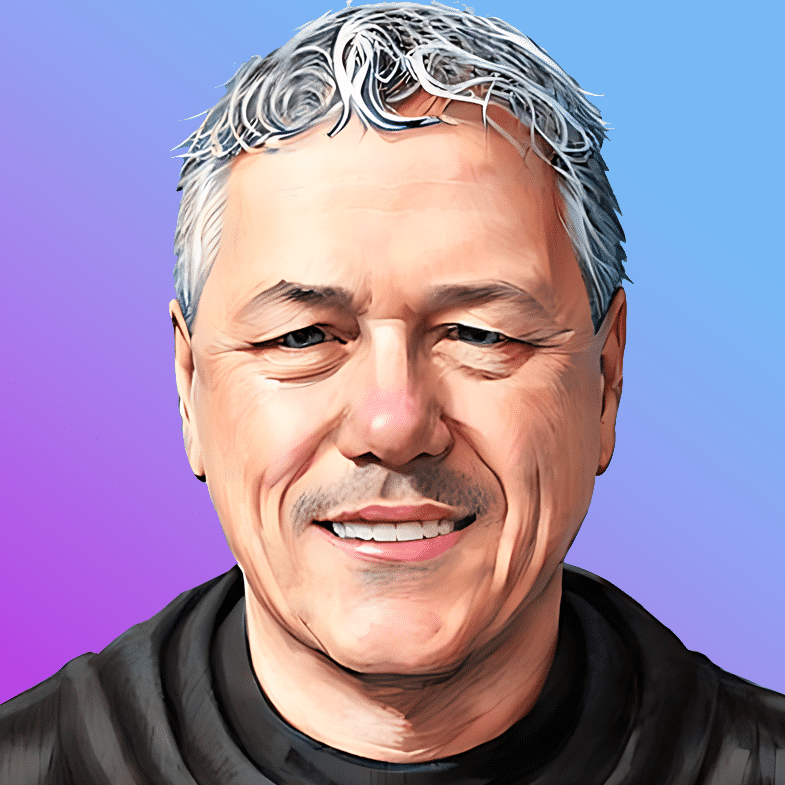
David Wilson
EVP, Digital Marketing and Strategy
David has more than 20 years working in digital marketing, covering in-house for a variety of companies, agencies and running his own digital marketing company. He has worked on Fortune 500 clients in the Pharmaceutical, CPG, Financial Services, and Healthcare verticals.
David brings a passion for proven results to the Zozimus digital marketing team. When asked what he likes about his job, David says that “every day his team has metrics that they are trying to hit for clients. At midnight the scoreboard gets set back to zero and we either hit our goals or we didn’t.”
BOSTON, MARS